Post History
#7: Post edited
How would you vaticinate to $-w_k$ from both sides of $w_{k + 1} = \dfrac{w_k - (1 - p)w_{k - 1}}{p}$??
- How would you vaticinate to $-w_k$ from both sides of $w_{k + 1} = \dfrac{w_k - (1 - p)w_{k - 1}}{p}$?
- This question appeared on my pop quiz last week. I got 0%. I achieved everything until the green equation, then I didn't know how to proceed. After reading this solution, I see that you must isolate $pw_{k + 1}$ and move $w_k$
- to the right.
- $\color{limegreen}{w_k = pw_{k + 1} + (1- p)w_{k - 1}} \iff pw_{k + 1} = w_k - (1 - p)w_{k - 1}$.
Then you must divide the equation by p.- $w_{k + 1} = \dfrac{w_k - (1 - p)w_{k - 1}}{p}$
Finally, you must subtract ${\color{red}{-w_k}}$ from both sides!- $w_{k + 1} {\color{red}{-w_k}} = \dfrac{1\color{red}{-p}}{p}(w_k - w_{k - 1})$
- These steps eluded me, are too tricky, and appear to come of the blue! Can you please naturalize (make natural) them? How would you progonosticate this algebra?
- 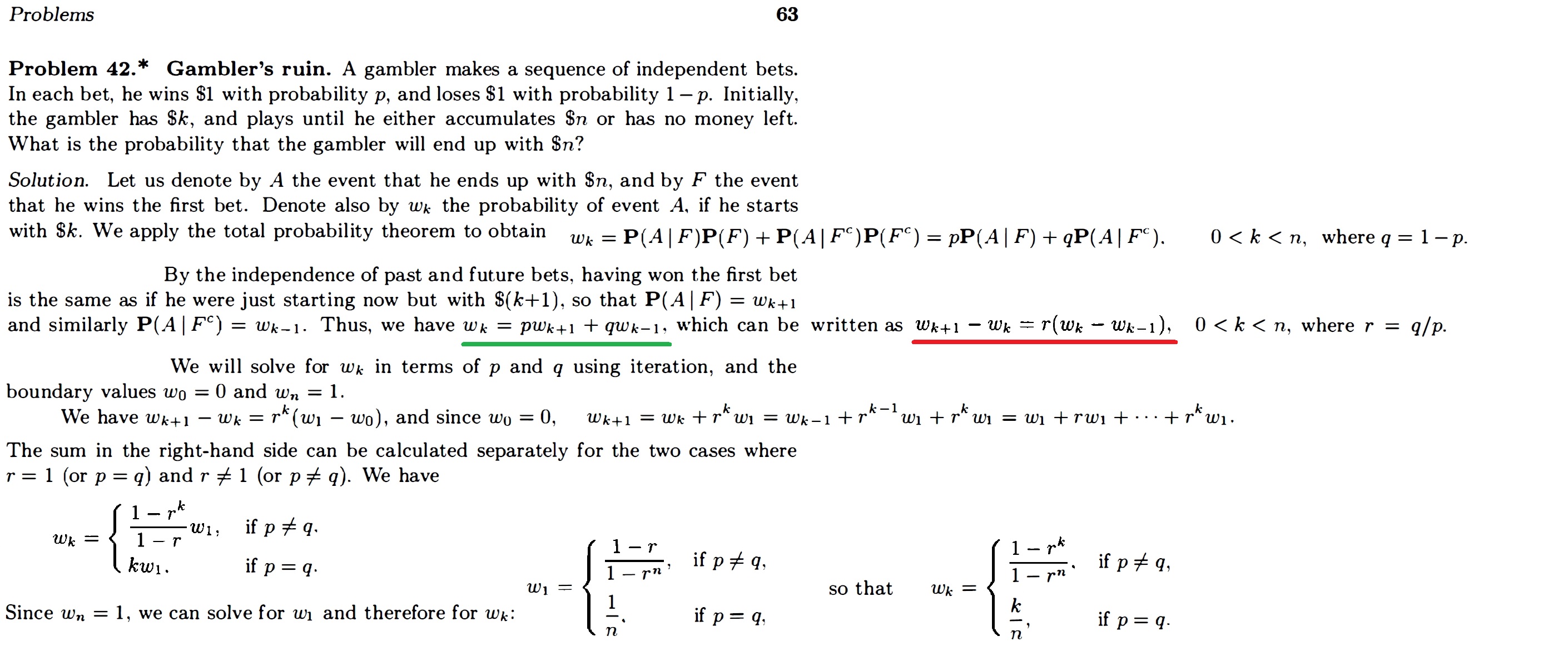
- Tsitsiklis, *Introduction to Probability* (2008 2e), p 63.
- This question appeared on my pop quiz last week. I got 0%. I achieved everything until the green equation, then I didn't know how to proceed. After reading this solution, I see that you must isolate $pw_{k + 1}$ and move $w_k$
- to the right.
- $\color{limegreen}{w_k = pw_{k + 1} + (1- p)w_{k - 1}} \iff pw_{k + 1} = w_k - (1 - p)w_{k - 1}$.
- 1. Then you must divide the equation by p.
- $w_{k + 1} = \dfrac{w_k - (1 - p)w_{k - 1}}{p}$
- 2. Finally, you must ${\color{red}{-w_k}}$ from both sides!
- $w_{k + 1} {\color{red}{-w_k}} = \dfrac{1\color{red}{-p}}{p}(w_k - w_{k - 1})$
- These steps eluded me, are too tricky, and appear to come of the blue! Can you please naturalize (make natural) them? How would you progonosticate this algebra?
- 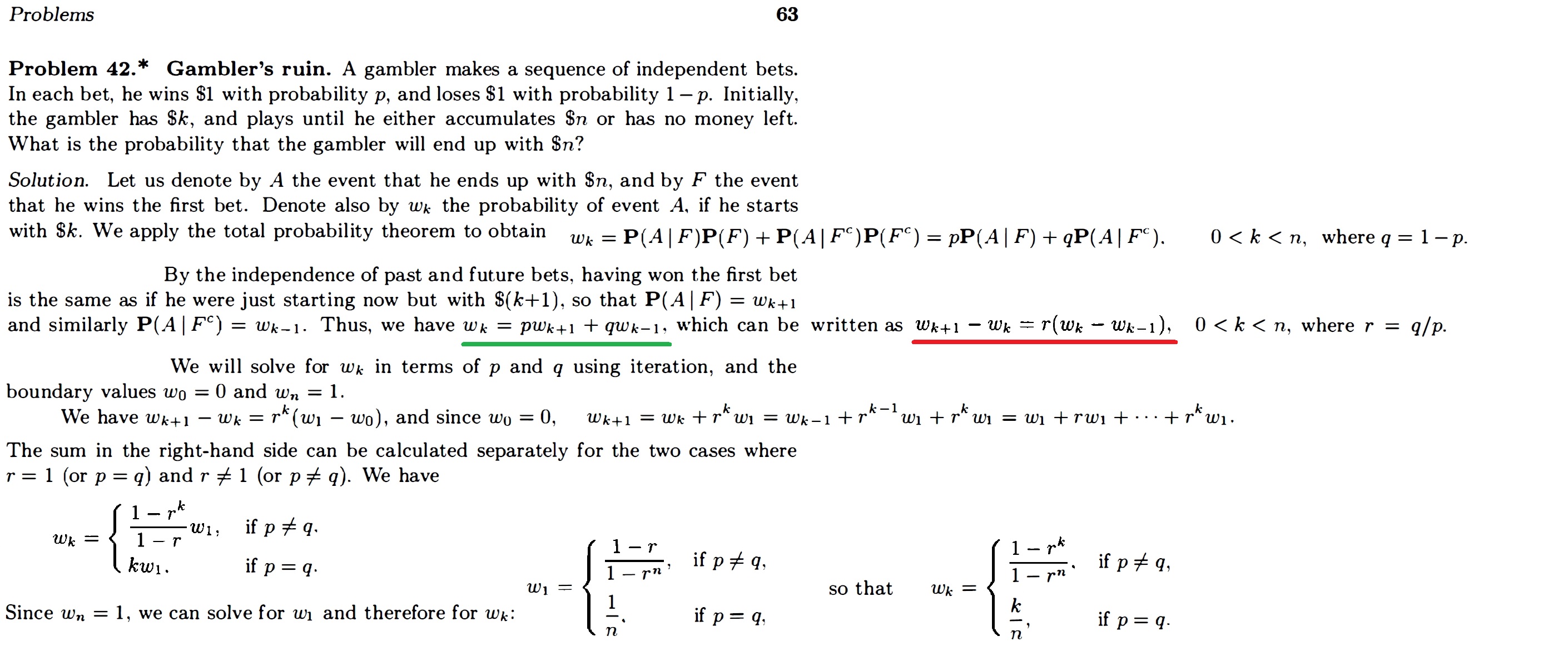
- Tsitsiklis, *Introduction to Probability* (2008 2e), p 63.
#6: Post edited
How would you vaticinate to $-w_k$ from both sides of $w_k = pw_{k + 1} + qw_{k - 1}$?
- How would you vaticinate to $-w_k$ from both sides of $w_{k + 1} = \dfrac{w_k - (1 - p)w_{k - 1}}{p}$??
#5: Post edited
How would you vaticinate to subtract $-w_k$ from both sides of $w_k = pw_{k + 1} + qw_{k - 1}$?
- How would you vaticinate to $-w_k$ from both sides of $w_k = pw_{k + 1} + qw_{k - 1}$?
#4: Post edited
This question appeared on my pop quiz last week. I got 0%. I did everything until the equality in green, and I didn't know how to proceed. After reading this solution, I see that you must isolate $pw_{k + 1}$ and move $w_k$- to the right.
- $\color{limegreen}{w_k = pw_{k + 1} + (1- p)w_{k - 1}} \iff pw_{k + 1} = w_k - (1 - p)w_{k - 1}$.
- Then you must divide the equation by p.
- $w_{k + 1} = \dfrac{w_k - (1 - p)w_{k - 1}}{p}$
- Finally, you must subtract ${\color{red}{-w_k}}$ from both sides!
- $w_{k + 1} {\color{red}{-w_k}} = \dfrac{1\color{red}{-p}}{p}(w_k - w_{k - 1})$
- These steps eluded me, are too tricky, and appear to come of the blue! Can you please naturalize (make natural) them? How would you progonosticate this algebra?
- 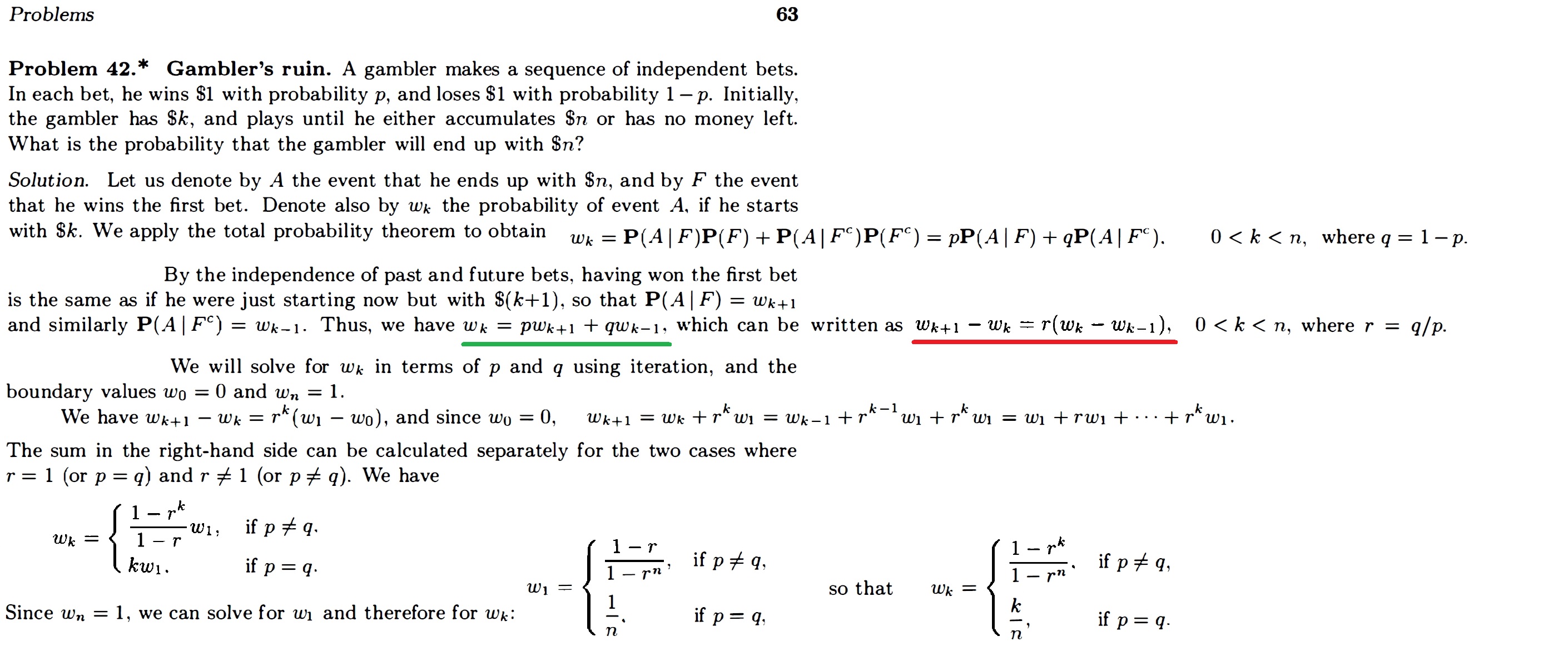
- Tsitsiklis, *Introduction to Probability* (2008 2e), p 63.
- This question appeared on my pop quiz last week. I got 0%. I achieved everything until the green equation, then I didn't know how to proceed. After reading this solution, I see that you must isolate $pw_{k + 1}$ and move $w_k$
- to the right.
- $\color{limegreen}{w_k = pw_{k + 1} + (1- p)w_{k - 1}} \iff pw_{k + 1} = w_k - (1 - p)w_{k - 1}$.
- Then you must divide the equation by p.
- $w_{k + 1} = \dfrac{w_k - (1 - p)w_{k - 1}}{p}$
- Finally, you must subtract ${\color{red}{-w_k}}$ from both sides!
- $w_{k + 1} {\color{red}{-w_k}} = \dfrac{1\color{red}{-p}}{p}(w_k - w_{k - 1})$
- These steps eluded me, are too tricky, and appear to come of the blue! Can you please naturalize (make natural) them? How would you progonosticate this algebra?
- 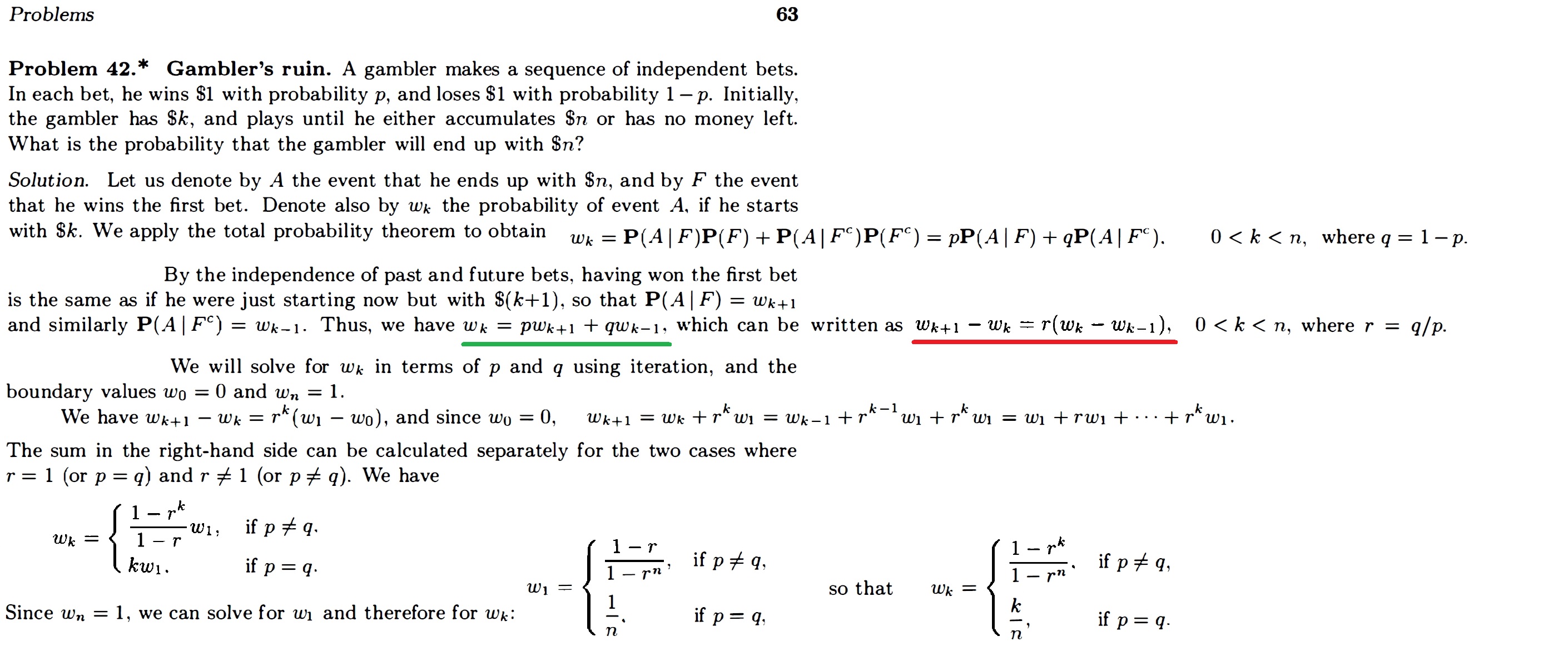
- Tsitsiklis, *Introduction to Probability* (2008 2e), p 63.
#3: Post edited
What did I bungle in calculating $P(D|+)$ with the Odds form of Bayes's Theorem?
- How would you vaticinate to subtract $-w_k$ from both sides of $w_k = pw_{k + 1} + qw_{k - 1}$?
Abbreviate D for Disease and + for a positive test. In [Prateek Karkare (Bachelor's, Masters, from Indian Institute of Technology, Bombay](https://www.linkedin.com/in/prateekkarkare/?originalSubdomain=in)'s [example](https://medium.com/x8-the-ai-community/a-simple-introduction-to-naive-bayes-23538a0395a), P(D) = 0.001, and P(+|D) = 0.99. I revised his explanation and chart.If there are 100,000 people, 100 people are diseased disease and the rest 99,900 are healthy. If these 100 sufferers get tested, $\color{green}{99}$ would test positive and $\color{red}{1}$ test negative. But what we overlook is that if the 99,900 healthy get tested, 1% (that is $\color{#e68a00}{999}$) will test false positive.If you test positive, then for you to be diseased, you must be $1$ of the $\color{green}{99}$ diseased people who tested positive. The total number of persons who tested positive is $\color{green}{99}+\color{#e68a00}{999}$. So the probability that you're diseased when you tested positive is $\dfrac{\color{green}{99}}{\color{green}{99}+\color{#e68a00}{999}} = 0.0901$.I tried to compute this [with odds](https://arbital.com/p/bayes_rule/?l=693) in green, but why's my answer wrong?>In the screened population, there's 1 sick patient for 4 [$\color{limegreen}{999}$] healthy patients. Sick patients are 3 [$\color{limegreen}{\dfrac{Pr(+|D)}{Pr(+|D^C)} = \dfrac{0.99}{0.01} = 99}$] times more likely to turn the tongue depressor black than healthy patients. $(1:4) \dot (3:1)=(3:4) \quad [\color{limegreen}{(1:999) \dot (99:1) = 1:111}]$ or 3 [$\color{limegreen}{1}$] sick patients to 4 [$\color{limegreen}{111}$] healthy patients among those that turn the tongue depressor black, corresponding to a probability of 3/7=43% [$\color{limegreen}{1/112 = 0.00893}$] that the patient is sick.
- This question appeared on my pop quiz last week. I got 0%. I did everything until the equality in green, and I didn't know how to proceed. After reading this solution, I see that you must isolate $pw_{k + 1}$ and move $w_k$
- to the right.
- $\color{limegreen}{w_k = pw_{k + 1} + (1- p)w_{k - 1}} \iff pw_{k + 1} = w_k - (1 - p)w_{k - 1}$.
- Then you must divide the equation by p.
- $w_{k + 1} = \dfrac{w_k - (1 - p)w_{k - 1}}{p}$
- Finally, you must subtract ${\color{red}{-w_k}}$ from both sides!
- $w_{k + 1} {\color{red}{-w_k}} = \dfrac{1\color{red}{-p}}{p}(w_k - w_{k - 1})$
- These steps eluded me, are too tricky, and appear to come of the blue! Can you please naturalize (make natural) them? How would you progonosticate this algebra?
- 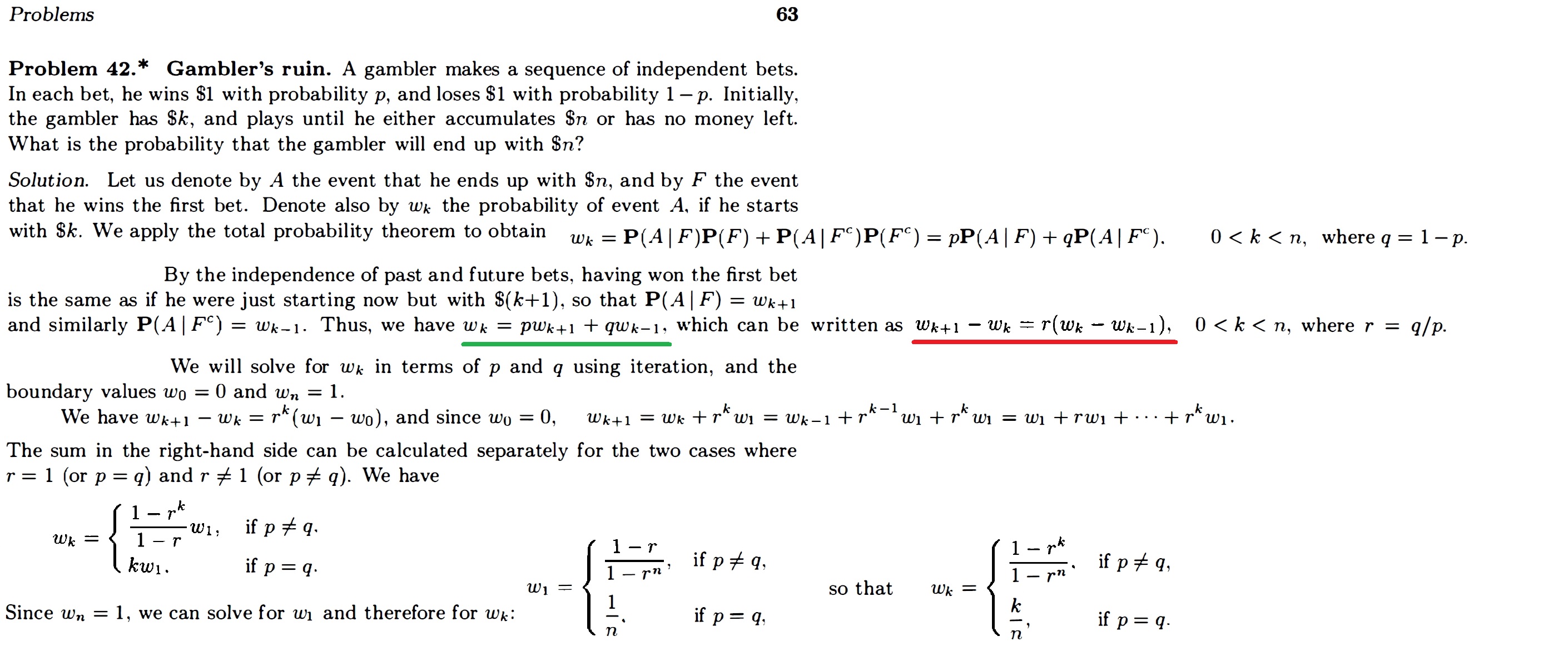
- Tsitsiklis, *Introduction to Probability* (2008 2e), p 63.
#2: Post edited
- Abbreviate D for Disease and + for a positive test. In [Prateek Karkare (Bachelor's, Masters, from Indian Institute of Technology, Bombay](https://www.linkedin.com/in/prateekkarkare/?originalSubdomain=in)'s [example](https://medium.com/x8-the-ai-community/a-simple-introduction-to-naive-bayes-23538a0395a), P(D) = 0.001, and P(+|D) = 0.99. I revised his explanation and chart.
- If there are 100,000 people, 100 people are diseased disease and the rest 99,900 are healthy. If these 100 sufferers get tested, $\color{green}{99}$ would test positive and $\color{red}{1}$ test negative. But what we overlook is that if the 99,900 healthy get tested, 1% (that is $\color{#e68a00}{999}$) will test false positive.
- If you test positive, then for you to be diseased, you must be $1$ of the $\color{green}{99}$ diseased people who tested positive. The total number of persons who tested positive is $\color{green}{99}+\color{#e68a00}{999}$. So the probability that you're diseased when you tested positive is $\dfrac{\color{green}{99}}{\color{green}{99}+\color{#e68a00}{999}} = 0.0901$.
- 
I tried to analyse this [with odds](https://arbital.com/p/bayes_rule/?l=693) in green, but my answer's wrong. What did I flub?- >In the screened population, there's 1 sick patient for 4 [$\color{limegreen}{999}$] healthy patients. Sick patients are 3 [$\color{limegreen}{\dfrac{Pr(+|D)}{Pr(+|D^C)} = \dfrac{0.99}{0.01} = 99}$] times more likely to turn the tongue depressor black than healthy patients. $(1:4) \dot (3:1)=(3:4) \quad [\color{limegreen}{(1:999) \dot (99:1) = 1:111}]$ or 3 [$\color{limegreen}{1}$] sick patients to 4 [$\color{limegreen}{111}$] healthy patients among those that turn the tongue depressor black, corresponding to a probability of 3/7=43% [$\color{limegreen}{1/112 = 0.00893}$] that the patient is sick.
- Abbreviate D for Disease and + for a positive test. In [Prateek Karkare (Bachelor's, Masters, from Indian Institute of Technology, Bombay](https://www.linkedin.com/in/prateekkarkare/?originalSubdomain=in)'s [example](https://medium.com/x8-the-ai-community/a-simple-introduction-to-naive-bayes-23538a0395a), P(D) = 0.001, and P(+|D) = 0.99. I revised his explanation and chart.
- If there are 100,000 people, 100 people are diseased disease and the rest 99,900 are healthy. If these 100 sufferers get tested, $\color{green}{99}$ would test positive and $\color{red}{1}$ test negative. But what we overlook is that if the 99,900 healthy get tested, 1% (that is $\color{#e68a00}{999}$) will test false positive.
- If you test positive, then for you to be diseased, you must be $1$ of the $\color{green}{99}$ diseased people who tested positive. The total number of persons who tested positive is $\color{green}{99}+\color{#e68a00}{999}$. So the probability that you're diseased when you tested positive is $\dfrac{\color{green}{99}}{\color{green}{99}+\color{#e68a00}{999}} = 0.0901$.
- 
- I tried to compute this [with odds](https://arbital.com/p/bayes_rule/?l=693) in green, but why's my answer wrong?
- >In the screened population, there's 1 sick patient for 4 [$\color{limegreen}{999}$] healthy patients. Sick patients are 3 [$\color{limegreen}{\dfrac{Pr(+|D)}{Pr(+|D^C)} = \dfrac{0.99}{0.01} = 99}$] times more likely to turn the tongue depressor black than healthy patients. $(1:4) \dot (3:1)=(3:4) \quad [\color{limegreen}{(1:999) \dot (99:1) = 1:111}]$ or 3 [$\color{limegreen}{1}$] sick patients to 4 [$\color{limegreen}{111}$] healthy patients among those that turn the tongue depressor black, corresponding to a probability of 3/7=43% [$\color{limegreen}{1/112 = 0.00893}$] that the patient is sick.
#1: Initial revision
What did I bungle in calculating $P(D|+)$ with the Odds form of Bayes's Theorem?
Abbreviate D for Disease and + for a positive test. In [Prateek Karkare (Bachelor's, Masters, from Indian Institute of Technology, Bombay](https://www.linkedin.com/in/prateekkarkare/?originalSubdomain=in)'s [example](https://medium.com/x8-the-ai-community/a-simple-introduction-to-naive-bayes-23538a0395a), P(D) = 0.001, and P(+|D) = 0.99. I revised his explanation and chart. If there are 100,000 people, 100 people are diseased disease and the rest 99,900 are healthy. If these 100 sufferers get tested, $\color{green}{99}$ would test positive and $\color{red}{1}$ test negative. But what we overlook is that if the 99,900 healthy get tested, 1% (that is $\color{#e68a00}{999}$) will test false positive. If you test positive, then for you to be diseased, you must be $1$ of the $\color{green}{99}$ diseased people who tested positive. The total number of persons who tested positive is $\color{green}{99}+\color{#e68a00}{999}$. So the probability that you're diseased when you tested positive is $\dfrac{\color{green}{99}}{\color{green}{99}+\color{#e68a00}{999}} = 0.0901$.  I tried to analyse this [with odds](https://arbital.com/p/bayes_rule/?l=693) in green, but my answer's wrong. What did I flub? >In the screened population, there's 1 sick patient for 4 [$\color{limegreen}{999}$] healthy patients. Sick patients are 3 [$\color{limegreen}{\dfrac{Pr(+|D)}{Pr(+|D^C)} = \dfrac{0.99}{0.01} = 99}$] times more likely to turn the tongue depressor black than healthy patients. $(1:4) \dot (3:1)=(3:4) \quad [\color{limegreen}{(1:999) \dot (99:1) = 1:111}]$ or 3 [$\color{limegreen}{1}$] sick patients to 4 [$\color{limegreen}{111}$] healthy patients among those that turn the tongue depressor black, corresponding to a probability of 3/7=43% [$\color{limegreen}{1/112 = 0.00893}$] that the patient is sick.